Продолжая использовать сайт, вы даете свое согласие на работу с этими файлами.
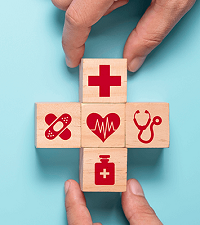
প্রিসিশন ও রিকল
প্যাটার্ন রিকগনেশন, ইনফরমেশন রিট্রিভাল ও বাইনারি ক্লাসিফিকেশন এ প্রিসিশন (পজেটিভ প্রোডাক্টিভ ভ্যালু) হলো অনেকগুলো ইন্সট্যান্সের মধ্য থেকে কাছাকাছি ইন্সট্যান্সের কিছু অংশ, অন্য দিকে রিকল (যেটি সেন্সিটিভিটি নামে পরিচিত) হলো কাছাকাছি ইন্সট্যান্সের অংশ যা সকল কাছাকাছি ইন্সট্যান্স থেকে বের করা হয়। প্রিসেশন ও রিকল দুটোই বাস্তবতার উপর অনুমান ও হিসাবের উপর গঠিত।
ধরুন, একটি কুকুর শনাক্ত করার কম্পিউটার প্রোগ্রামের কথা যেখানে অনেকগুলো ছবির মধ্যে প্রোগ্রামটি ১২টি কুকুরের মধ্যে ৮টি কুকুর শনাক্ত করল আর বাকিগুলো বিড়াল। অসলে, ৮টি কুকুরের মধ্যে সত্যিকার অর্থে ৫টি কুকুর (ট্রু পজেটিভ) ছিল বাকিগুলো বিড়াল (ফলস পজেটিভ)। তাহলে প্রোগ্রামের প্রিসিশন হবে ৫/৮ যেখানে রিকল হবে ৫/১২। যখন আমরা একটি সার্চ ইঞ্জিন সার্চ করি তখন ৩০টি পেজের মধ্যে ২০টি পেজ বিষয়বস্তু সংশ্লিষ্ট, অন্যদিকে অতিরিক্ত ৪০টি বিষয় সংশ্লিষ্ট পেজ আসতে ব্যর্থ হয়। তখন প্রিসিশন হবে ২০/৩০ যেখানে রিকল ২০/৬০ = ১/৩। কাজেই, প্রিসিশন হলো "সার্চ রেজাল্ট কত ভালো সেটা" আর রিকল হলো "সার্চ রেজাল্ট কয়টা দিতে পারলো সেটা"।
পরিসংখ্যান অনুযায়ী, যদি নাল হাইপোথেসিস হয় সমস্ত আইটেম তাহলে সেগুলো অসম্পর্কিত যেখানে হাইপোথেসিস সিম্পল সাইজের নির্ধারিত সংখ্যার উপর ভিত্তি করে সিদ্ধান্ত নেয়া হয় হাইপো থেসিসটি গৃহীত হবে নাকি বর্জিত হবে, টাইপ ওয়ান ও টাইপ টু ইরর (সফল ১০০% সেন্সিভিটি আর স্পেসিফিসিটি) গুলোতে তুলনামুলকভাবে ধরা হয় সফল প্রিসিশন (ফলস পজেটিভ নেই) এবং সফল প্রিসিশন (ফলস নেগেটিভ নেই)। উপরের প্যাটার্ন রিকগনিশনের উদাহরণ অনুযায়ী ৮ - ৫ = ৩ টাইপ ওয়ান ইরর এবং ১২ - ৫ = ৭ টাইপ টু ইরর। প্রিসিশনে গুনগত মান দেখা হ্য। সেন্সিটিভিট আর স্পেসিফিসিটির মধ্যে সম্পর্ক হলো প্রিসিশন মোটের উপর সম্ভব্য সম্ভব্যতার উপর নির্ভর করে।
সহজ কথায়, হাই প্রিসিশন মানে হলো যখন একটি অ্যালগরিদম দূরবর্তীর চেয়ে সবথেকে কাছাকাছি ফলাফল দেয়া, অন্যদিকে হাই রিকল মানে হলে একটি অ্যালগরিদম সবথেকে কাছাকাছি ফলাফল দেবে।
পরিচিতি
information retrieval (IR) চিত্রে, ইনস্ট্যান্সগুলো ডকুমেন্ট আর টাস্কটি উল্লিখিত সার্চ টার্মের কাছাকাছি অথবা সমান, ডকুমেন্টগুলোর একটি সেট রিটার্ন করে; প্রত্যেক ডকুমেন্টের দুটি ক্যাটাগরির একটিতে, হয় "কাছাকাছি" আর না হয় "কাছাকাছি নয়" এসাইন করে। In this case, the "relevant" documents are simply those that belong to the "relevant" category. Recall is defined as the number of relevant documents retrieved by a search divided by the total number of existing relevant documents, while precision is defined as the number of relevant documents retrieved by a search divided by the total number of documents retrieved by that search.
In a classification task, the precision for a class is the number of true positives (i.e. the number of items correctly labeled as belonging to the positive class) divided by the total number of elements labeled as belonging to the positive class (i.e. the sum of true positives and false positives, which are items incorrectly labeled as belonging to the class). Recall in this context is defined as the number of true positives divided by the total number of elements that actually belong to the positive class (i.e. the sum of true positives and false negatives, which are items which were not labeled as belonging to the positive class but should have been).
In information retrieval, a perfect precision score of 1.0 means that every result retrieved by a search was relevant (but says nothing about whether all relevant documents were retrieved) whereas a perfect recall score of 1.0 means that all relevant documents were retrieved by the search (but says nothing about how many irrelevant documents were also retrieved).
In a classification task, a precision score of 1.0 for a class C means that every item labeled as belonging to class C does indeed belong to class C (but says nothing about the number of items from class C that were not labeled correctly) whereas a recall of 1.0 means that every item from class C was labeled as belonging to class C (but says nothing about how many other items were incorrectly also labeled as belonging to class C).
Often, there is an inverse relationship between precision and recall, where it is possible to increase one at the cost of reducing the other. Brain surgery provides an illustrative example of the tradeoff. Consider a brain surgeon tasked with removing a cancerous tumor from a patient’s brain. The surgeon needs to remove all of the tumor cells since any remaining cancer cells will regenerate the tumor. Conversely, the surgeon must not remove healthy brain cells since that would leave the patient with impaired brain function. The surgeon may be more liberal in the area of the brain he removes to ensure he has extracted all the cancer cells. This decision increases recall but reduces precision. On the other hand, the surgeon may be more conservative in the brain he removes to ensure he extracts only cancer cells. This decision increases precision but reduces recall. That is to say, greater recall increases the chances of removing healthy cells (negative outcome) and increases the chances of removing all cancer cells (positive outcome). Greater precision decreases the chances of removing healthy cells (positive outcome) but also decreases the chances of removing all cancer cells (negative outcome).
Usually, precision and recall scores are not discussed in isolation. Instead, either values for one measure are compared for a fixed level at the other measure (e.g. precision at a recall level of 0.75) or both are combined into a single measure. Examples of measures that are a combination of precision and recall are the F-measure (the weighted harmonic mean of precision and recall), or the Matthews correlation coefficient, which is a geometric mean of the chance-corrected variants: the regression coefficients Informedness (DeltaP') and Markedness (DeltaP).Accuracy is a weighted arithmetic mean of Precision and Inverse Precision (weighted by Bias) as well as a weighted arithmetic mean of Recall and Inverse Recall (weighted by Prevalence). Inverse Precision and Inverse Recall are simply the Precision and Recall of the inverse problem where positive and negative labels are exchanged (for both real classes and prediction labels). Recall and Inverse Recall, or equivalently true positive rate and false positive rate, are frequently plotted against each other as ROC curves and provide a principled mechanism to explore operating point tradeoffs. Outside of Information Retrieval, the application of Recall, Precision and F-measure are argued to be flawed as they ignore the true negative cell of the contingency table, and they are easily manipulated by biasing the predictions. The first problem is 'solved' by using Accuracy and the second problem is 'solved' by discounting the chance component and renormalizing to Cohen's kappa, but this no longer affords the opportunity to explore tradeoffs graphically. However, Informedness and Markedness are Kappa-like renormalizations of Recall and Precision, and their geometric mean Matthews correlation coefficient thus acts like a debiased F-measure.
সংজ্ঞা (ইনফরমেশন রিট্রিভাল কনটেক্সট))
In information retrieval contexts, precision and recall are defined in terms of a set of retrieved documents (e.g. the list of documents produced by a web search engine for a query) and a set of relevant documents (e.g. the list of all documents on the internet that are relevant for a certain topic), cf. relevance. The measures were defined in Perry, Kent & Berry (1955).
প্রিসিশন
In the field of information retrieval, precision is the fraction of retrieved documents that are relevant to the query:
For example, for a text search on a set of documents, precision is the number of correct results divided by the number of all returned results.
Precision takes all retrieved documents into account, but it can also be evaluated at a given cut-off rank, considering only the topmost results returned by the system. This measure is called precision at n or P@n.
Precision is used with recall, the percent of all relevant documents that is returned by the search. The two measures are sometimes used together in the F1 Score (or f-measure) to provide a single measurement for a system.
Note that the meaning and usage of "precision" in the field of information retrieval differs from the definition of accuracy and precision within other branches of science and technology.
রিকল
In information retrieval, recall is the fraction of the relevant documents that are successfully retrieved.
For example, for a text search on a set of documents, recall is the number of correct results divided by the number of results that should have been returned.
In binary classification, recall is called sensitivity. It can be viewed as the probability that a relevant document is retrieved by the query.
It is trivial to achieve recall of 100% by returning all documents in response to any query. Therefore, recall alone is not enough but one needs to measure the number of non-relevant documents also, for example by also computing the precision.
সংজ্ঞা (classification context)
For classification tasks, the terms true positives, true negatives, false positives, and false negatives (see Type I and type II errors for definitions) compare the results of the classifier under test with trusted external judgments. The terms positive and negative refer to the classifier's prediction (sometimes known as the expectation), and the terms true and false refer to whether that prediction corresponds to the external judgment (sometimes known as the observation).
Let us define an experiment from P positive instances and N negative instances for some condition. The four outcomes can be formulated in a 2×2 contingency table or confusion matrix, as follows:
টেমপ্লেট:DiagnosticTesting Diagram টেমপ্লেট:Confusion matrix terms
- Baeza-Yates, Ricardo; Ribeiro-Neto, Berthier (1999). Modern Information Retrieval. New York, NY: ACM Press, Addison-Wesley, Seiten 75 ff. আইএসবিএন ০-২০১-৩৯৮২৯-X
- Hjørland, Birger (2010); The foundation of the concept of relevance, Journal of the American Society for Information Science and Technology, 61(2), 217-237
- Makhoul, John; Kubala, Francis; Schwartz, Richard; and Weischedel, Ralph (1999); Performance measures for information extraction, in Proceedings of DARPA Broadcast News Workshop, Herndon, VA, February 1999
- Perry, James W.; Kent, Allen; Berry, Madeline M. (১৯৫৫)। "Machine literature searching X. Machine language; factors underlying its design and development"। American Documentation। 6 (4): 242। ডিওআই:10.1002/asi.5090060411।
- van Rijsbergen, Cornelis Joost "Keith" (1979); Information Retrieval, London, GB; Boston, MA: Butterworth, 2nd Edition, আইএসবিএন ০-৪০৮-৭০৯২৯-৪